Cloud computing and ai have combined to fuel each other's stunning growth
Technology leaps would be impossible without cloud computing. That's because AI requires data, and lots of it.
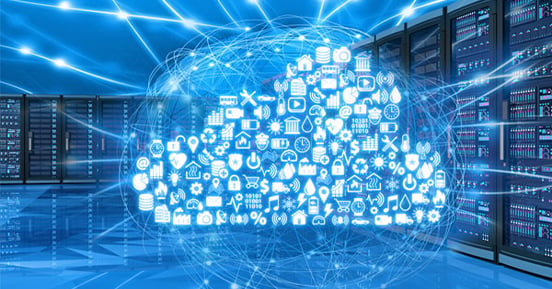
Artificial intelligence research used to be limited to people who could afford the world's most powerful computers. Today, that's everybody.
The convergence of fast computers, inexpensive storage and free software has enabled recent breathtaking advancement in artificial intelligence (AI) software, but the combination of cloud computing and AI has been the most important driving force.
Technological advances like voice recognition, self-driving vehicles and automated document classification would have been impossible without cloud computing. That's because AI requires data, and lots of it. Not long ago, none but the largest organizations could amass the data volumes necessary to train AI algorithms, but thanks to cloud computing, those barriers have fallen away.
The More Data the Better: Amassing, Sifting and Learning
The two most common applications of AI are machine learning and deep learning. Machine learning is used to sort through large amounts of data to identify patterns that wouldn't be obvious to a human observer. For example, it can ingest millions of patient records to find adverse drug interactions or suggest purchases to shoppers based upon the buying preferences of similar customers.
Deep learning uses a neural network structure like the human brain to develop deep knowledge about a specific domain. Among its most popular uses are voice and image recognition.
Both use cases require the algorithms to be "trained," which involves sifting through large databases repeatedly to spot and reinforce patterns. The more data the better. That's where the cloud has played a huge role.
Consider cloud-based voice-activated assistants like Amazon Echo and Google Assistant, which are now everywhere. They can recognize — without training — male, female and children's voices and a wide variety of accents. The reason is that the software is constantly trained on a database of all the commands that have been captured over a period of years. This data is gathered and stored in the cloud, where it serves as a continually improving knowledge base for deep learning. The more people use voice-activated assistants, the smarter they get.
That data also serves as a resource to machine learning algorithms that enable the assistants to interpret commands. The reason Google Assistant knows that "tell me when I'm going to get there" and "what's my ETA?" are equivalent requests is that it has sorted through billions of commands to determine which ones are similar.
Accessible Cloud Processing Opens Doors
But the cloud's contributions to AI's ascendancy go beyond just data. Cloud computing has also brought the tools and training needed to develop AI applications out of university classrooms and into the open market. Powerful processing engines optimized for AI applications can be rented in the cloud on an hourly basis. These multimillion-dollar machines were previously inaccessible to all but government research labs and major corporations.
The major cloud providers also support a variety of machine and deep learning software frameworks, which greatly simplify the process of building applications. Developers can share models, improve upon each other's success and swap solutions to their problems in cloud community forums. The benefits of an open-sharing and collaboration environment are one reason about 70% of enterprise AI development will be done in the cloud this year, according to Deloitte Global. A survey by LogicMonitor concluded that AI will drive two-thirds of public cloud use by 2020.
Better Algorithms Mean Better Results
Finally, the cloud serves as a critical distribution mechanism for training data, which is needed to ensure the quality of AI applications. Machine learning algorithms, in particular, are only as good as the data they're given. If training data is incomplete or biased, the algorithm may deliver misleading results or even amplify the bias in the source data.
High-quality training data sets are needed to ensure that models are robust and accurate. The cloud has made a cornucopia of training data available from corporations, nonprofits and government entities, much of it at no charge, according to Data Driven Investor. It also provides a means for commercial data collection firms to distribute up-to-the-minute information to their clients for training in real time.
AI has long been kept from reaching its potential by the intensive processing and data resources it demands. As the world's largest computing resource, the cloud has erased those barriers, effectively making the magic of thinking machines available to everyone.
The convergence of fast computers, inexpensive storage and free software has enabled recent breathtaking advancement in artificial intelligence (AI) software, but the combination of cloud computing and AI has been the most important driving force.
Technological advances like voice recognition, self-driving vehicles and automated document classification would have been impossible without cloud computing. That's because AI requires data, and lots of it. Not long ago, none but the largest organizations could amass the data volumes necessary to train AI algorithms, but thanks to cloud computing, those barriers have fallen away.
The More Data the Better: Amassing, Sifting and Learning
The two most common applications of AI are machine learning and deep learning. Machine learning is used to sort through large amounts of data to identify patterns that wouldn't be obvious to a human observer. For example, it can ingest millions of patient records to find adverse drug interactions or suggest purchases to shoppers based upon the buying preferences of similar customers.
Deep learning uses a neural network structure like the human brain to develop deep knowledge about a specific domain. Among its most popular uses are voice and image recognition.
Both use cases require the algorithms to be "trained," which involves sifting through large databases repeatedly to spot and reinforce patterns. The more data the better. That's where the cloud has played a huge role.
Consider cloud-based voice-activated assistants like Amazon Echo and Google Assistant, which are now everywhere. They can recognize — without training — male, female and children's voices and a wide variety of accents. The reason is that the software is constantly trained on a database of all the commands that have been captured over a period of years. This data is gathered and stored in the cloud, where it serves as a continually improving knowledge base for deep learning. The more people use voice-activated assistants, the smarter they get.
That data also serves as a resource to machine learning algorithms that enable the assistants to interpret commands. The reason Google Assistant knows that "tell me when I'm going to get there" and "what's my ETA?" are equivalent requests is that it has sorted through billions of commands to determine which ones are similar.
Accessible Cloud Processing Opens Doors
But the cloud's contributions to AI's ascendancy go beyond just data. Cloud computing has also brought the tools and training needed to develop AI applications out of university classrooms and into the open market. Powerful processing engines optimized for AI applications can be rented in the cloud on an hourly basis. These multimillion-dollar machines were previously inaccessible to all but government research labs and major corporations.
The major cloud providers also support a variety of machine and deep learning software frameworks, which greatly simplify the process of building applications. Developers can share models, improve upon each other's success and swap solutions to their problems in cloud community forums. The benefits of an open-sharing and collaboration environment are one reason about 70% of enterprise AI development will be done in the cloud this year, according to Deloitte Global. A survey by LogicMonitor concluded that AI will drive two-thirds of public cloud use by 2020.
Better Algorithms Mean Better Results
Finally, the cloud serves as a critical distribution mechanism for training data, which is needed to ensure the quality of AI applications. Machine learning algorithms, in particular, are only as good as the data they're given. If training data is incomplete or biased, the algorithm may deliver misleading results or even amplify the bias in the source data.
High-quality training data sets are needed to ensure that models are robust and accurate. The cloud has made a cornucopia of training data available from corporations, nonprofits and government entities, much of it at no charge, according to Data Driven Investor. It also provides a means for commercial data collection firms to distribute up-to-the-minute information to their clients for training in real time.
AI has long been kept from reaching its potential by the intensive processing and data resources it demands. As the world's largest computing resource, the cloud has erased those barriers, effectively making the magic of thinking machines available to everyone.
Related resources
View More Resources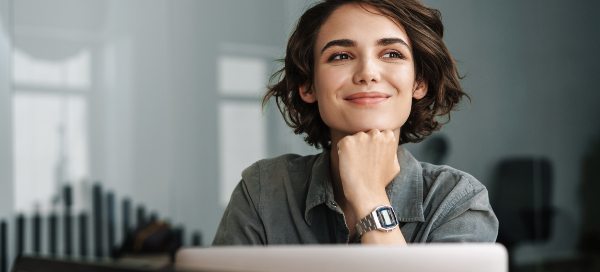