Competitive advantages of implementing artificial intelligence in banking
Find out how implementing artificial intelligence in banking can help unlock valuable customer data and insights.
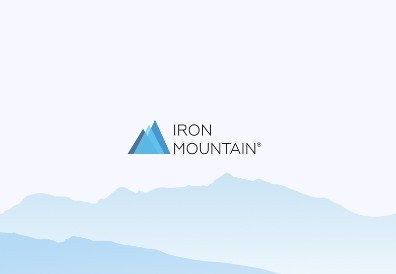
Financial firms that act quickly to apply artificial intelligence tools will gain a significant competitive edge.
Nearly 80% of the information captured during the processing of a mortgage loan is in forms that – until recently – have been all but inaccessible to computers. This so-called unstructured data such as emails, phone calls, text documents and images could have great value to financial institutions in helping them better understand customers, assess risk and create new products, but there simply aren’t enough people to read and analyze so much information.
That’s changing, however, thanks to two artificial intelligence-related disciplines called natural language processing (NLP) and machine learning (ML). These companion technologies enable computers to sort through vast amounts of unstructured data to identify patterns that can yield valuable insights that would elude even human analysts, who are limited in the amount of data they can absorb. NLP and ML are already speeding up loan and mortgage application processing, but the more important dividend will be the customer insights they deliver that can help bankers make better decisions.
On the efficiency front, AI can scan documents to look for missing or mis-categorized information and immediately notify applicants of the need for corrections, reducing delays and rework. Machines can also create descriptions of information inside documents, emails and recordings – a type of label called “metadata” – that makes the content easily searchable. That means employees can more quickly find the information they need.
The more intriguing potential of ML and NLP, though, is to better understand customers. For example, emails and phone calls can be analyzed for language that can indicates extreme satisfaction or dissatisfaction. Happy customers can thus be presented with rewards or new product options while angry customers are immediately connected with a sympathetic employee who helps relieve their frustration. Machine learning programs can also comb through large amounts of customer interaction data to identify areas of common concern, helping the bank to prioritize its investments.
The same technology can be used to learn more about what customers want. For example, a bank could mine social media conversations to identify customers who are struggling with eldercare costs or about to send a child to college and reach out with customized loan offers. Or it could scour a customer’s account records to see where that person frequently does business and reach out with an offer.
Conversely, machine learning algorithms can identify patterns of behavior that point to potentially fraudulent activity or raise warning flags about a person’s credit-worthiness. That lowers risk, enabling the institution to invest in better products and services.
The goal is ultimately to get to “markets of one,” in which each customer’s experience is unique and tailored to his or her interests.
Much of what we wish we knew about customers is currently locked up in forms that are impossible or impractical for humans to process. Those barriers are quickly falling, though. Financial firms that act quickly to apply these new tools will gain a significant competitive edge.
Nearly 80% of the information captured during the processing of a mortgage loan is in forms that – until recently – have been all but inaccessible to computers. This so-called unstructured data such as emails, phone calls, text documents and images could have great value to financial institutions in helping them better understand customers, assess risk and create new products, but there simply aren’t enough people to read and analyze so much information.
That’s changing, however, thanks to two artificial intelligence-related disciplines called natural language processing (NLP) and machine learning (ML). These companion technologies enable computers to sort through vast amounts of unstructured data to identify patterns that can yield valuable insights that would elude even human analysts, who are limited in the amount of data they can absorb. NLP and ML are already speeding up loan and mortgage application processing, but the more important dividend will be the customer insights they deliver that can help bankers make better decisions.
On the efficiency front, AI can scan documents to look for missing or mis-categorized information and immediately notify applicants of the need for corrections, reducing delays and rework. Machines can also create descriptions of information inside documents, emails and recordings – a type of label called “metadata” – that makes the content easily searchable. That means employees can more quickly find the information they need.
The more intriguing potential of ML and NLP, though, is to better understand customers. For example, emails and phone calls can be analyzed for language that can indicates extreme satisfaction or dissatisfaction. Happy customers can thus be presented with rewards or new product options while angry customers are immediately connected with a sympathetic employee who helps relieve their frustration. Machine learning programs can also comb through large amounts of customer interaction data to identify areas of common concern, helping the bank to prioritize its investments.
The same technology can be used to learn more about what customers want. For example, a bank could mine social media conversations to identify customers who are struggling with eldercare costs or about to send a child to college and reach out with customized loan offers. Or it could scour a customer’s account records to see where that person frequently does business and reach out with an offer.
Conversely, machine learning algorithms can identify patterns of behavior that point to potentially fraudulent activity or raise warning flags about a person’s credit-worthiness. That lowers risk, enabling the institution to invest in better products and services.
The goal is ultimately to get to “markets of one,” in which each customer’s experience is unique and tailored to his or her interests.
Much of what we wish we knew about customers is currently locked up in forms that are impossible or impractical for humans to process. Those barriers are quickly falling, though. Financial firms that act quickly to apply these new tools will gain a significant competitive edge.
In terms of efficiency, AI can scan documents to look for missing or mis-categorised information. It can immediately notify applicants of the need for corrections, reducing delays. Machines can also create descriptions of information inside documents, emails and recordings – a type of label called "metadata" - that makes the content easily searchable. This means employees can more quickly find the information they need.
The more intriguing potential of ML and NLP, though, is to better understand customers. For example, emails and phone calls can be analysed for language that can indicate extreme satisfaction or dissatisfaction. Happy customers can then be presented with rewards or new product options while angry customers are immediately connected with a sympathetic employee who helps relieve their frustration. Machine-learning programs can also comb through large amounts of customer-interaction data to identify areas of common concern, helping the bank to prioritise its investments.
The same technology can be used to learn more about what customers want. For example, a bank could mine social media conversations to identify customers who are struggling with utility costs or about to send a child to university and reach out with customised loan offers. Or it could scour a customer's account records to see where that person frequently does business and reach out with an offer.
Conversely, machine-learning algorithms can identify patterns of behaviour that point to potentially fraudulent activity or raise warning flags about a person's credit-worthiness. That lowers risk, enabling the institution to invest in better products and services.
The goal is ultimately to get to "markets of one", in which each customer's experience is unique and tailored to his or her interests.
Much of what we wish we knew about customers is currently locked up in forms that are impossible or impractical for humans to process. Those barriers are quickly falling, though. Financial firms that act quickly to apply these new tools will gain a significant competitive edge.
To learn more, read the white paper on Data Search and Discovery in Banking
Related resources
View More Resources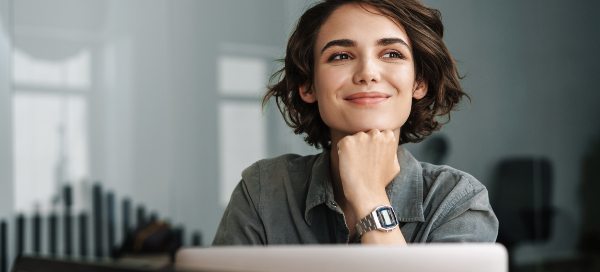